Ahmed Hosny
Data Scientist
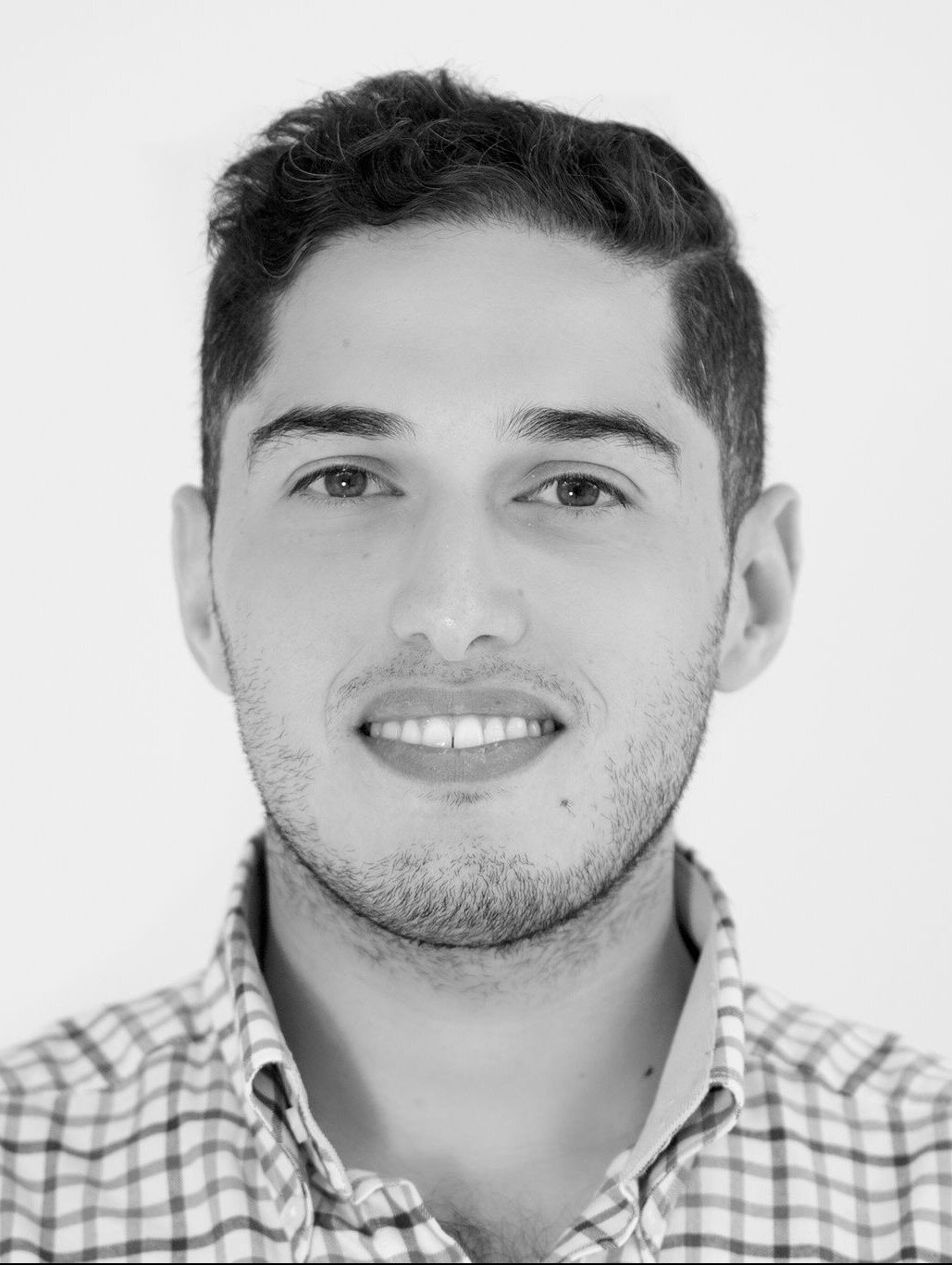
I am currently a data scientist at the Dana-Farber Cancer Institute, Brigham and Women’s Hospital. My research explores capturing tumor phenotypes through computer vision, machine learning and quantitative shape descriptors. I am also a research affiliate at MIT Media Lab’s Mediated Matter group working on mechanical characterizations of 3d printed materials. Previously a research fellow at Harvard’s Wyss Institute for Biologically Inspired Engineering, I studied geometry-function relationships in biological composites and fabricated their synthetic analogs. I also worked at Brigham and Women’s hospital’s Surgical Planning Lab designing hardware for sizing heart valve prosthesis and image guided surgery. My interests include computational geometry, additive manufacturing and developing tools for the web. I received a Master’s degree in Design Technology from the Harvard Graduate School of Design with thesis in Material Analytics bridging solid mechanics and machine learning. With 4 years of industry experience as an architect, I have been involved with Foster + Partners in Beijing and Playze in Shanghai working on multiple construction projects. I also worked in New York, Dubai & Abu Dhabi with offices including Case, Aedas and BurtHill. I received a Bachelor of Architecture from the American University of Sharjah in 2009. On weekends, I enjoy flying single engine planes, practicing Mandarin and eating chocolate chip cookies.
email: ahmed_hosny@dfci.harvard.edu
Research Highlights
In Lancet Digital Health, we published a clinical validation of deep learning algorithms to target lung cancer tumors.
In Cancer Cell, we published our perspective on the impact of AI in Clinical Oncology.
Scientific American discusses the hype in AI and highlights study from AIM.
MIT Technology Review highlights our article published in Nature about transparent AI.
AIM investigators describe their view on AI reproducibility and transparency.
In Nature Reviews Clinical Oncology, we highlight how AI is transforming the field of radiation oncology to treat patients more accurately and efficiently.
In a news item, Scientific American discusses our deep learning study to automatically predict mortality from x-rays.
In a special report about innovations in AI and digital health, Nature highlights two studies from AIM investigators.
Science Magazine published our perspective on the application of Artificial intelligence in resource-poor health care settings.
Our article "Deep learning for lung cancer prognostication" was selected by IMIA as one of the best articles of the year.
JAMA Network Open published our study about using deep learning to extract prognostic information from chest radiographs
Clinical Cancer Research published about deep learning applied to serial imaging to improve outcome predictions.
Our publication about artificial intelligence in cancer imaging was highlighted on the cover of CA: A Cancer Journal for clinicians.
A review of AI applications in the imaging of several tumor types has been published in CA: A Cancer Journal for clinicians.
AIM’s publication “AI in Radiology” was featured in the Nature about promising technologies transforming the world.
Our publication “Artificial Intelligence in Radiology” was highlighted on the cover of Nature Reviews Cancer.
Nature Reviews Cancer published our opinion article on the application of artificial intelligence to image-based tasks in the field of radiology.
PLOS Medicine published our study exploring deep learning for predicting overall survival in lung cancer patients.
Clinical Cancer Research published our article outlining best practices for analyzing medical imaging data using AI.