Michael Lu
Faculty Member
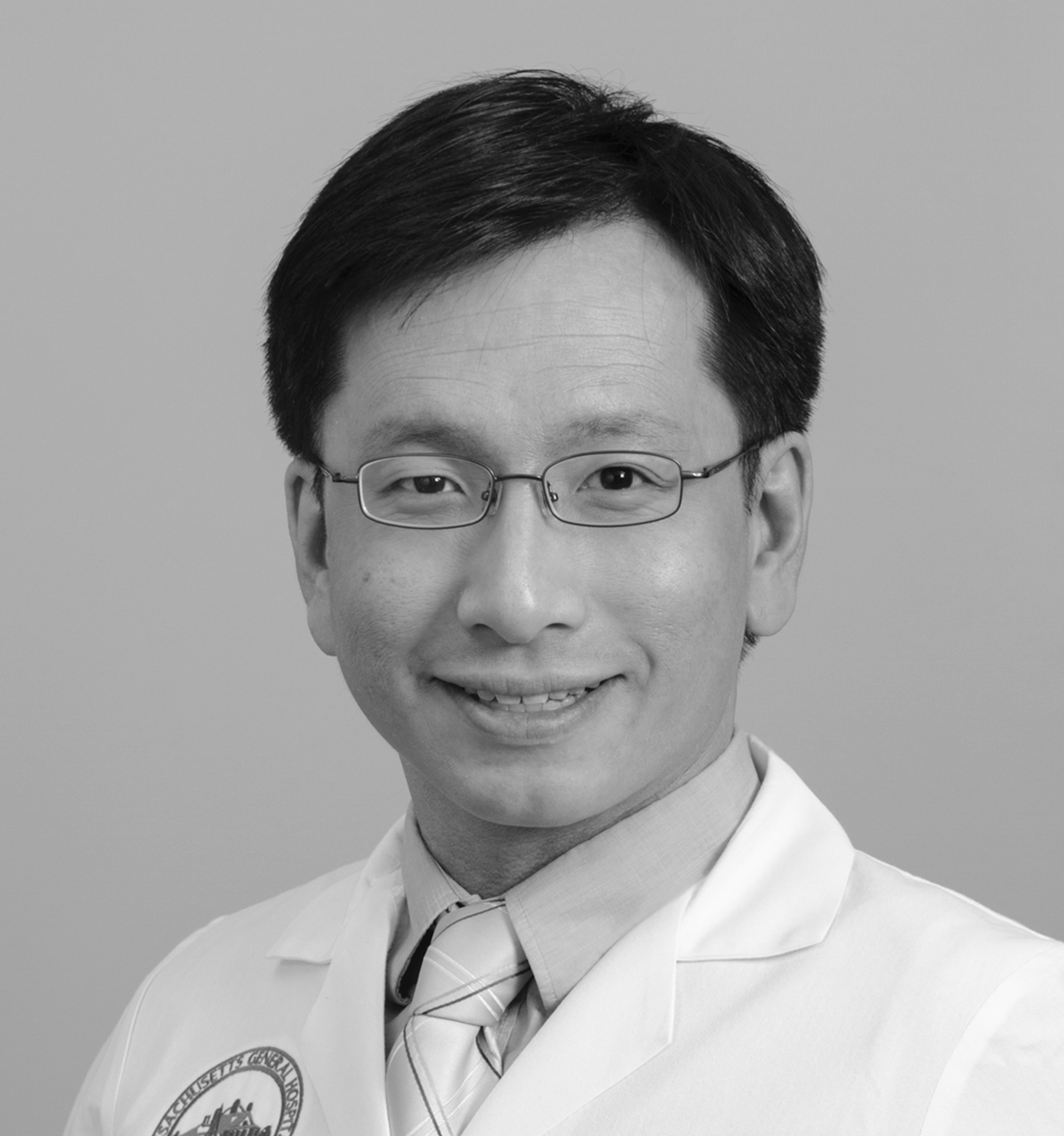
Michael T. Lu, MD, MPH, is Director of Artificial Intelligence at the Massachusetts General Hospital (MGH) Cardiovascular Imaging Research Center (CIRC), Director of Research at the MGH Division of Cardiovascular Imaging, and Assistant Professor of Radiology at Harvard Medical School.
Dr. Lu’s research focuses on A) machine learning to predict health outcomes from imaging and B) clinical trials using CT to improve health. Recent work has used convolutional neural networks to predict longevity and cancer from routine chest radiograph images. He co-chairs the Mechanistic CT Substudy of REPRIEVE, a NHLBI-sponsored multicenter randomized controlled trial of statins to reduce coronary plaque and prevent cardiovascular events in HIV. His research has been supported by the American Heart Association, American Roentgen Ray Society, American College of Radiology, MGH Fund for Medical Discovery, and Harvard University Center for AIDS Research.
Dr. Lu earned his undergraduate, MD, and MPH degrees from Harvard University. His training consisted of a transitional internship in Surgery and Medicine at Newton-Wellesley Hospital, residency in Diagnostic Radiology at the University of California, San Francisco, fellowship in Thoracic and Cardiac Imaging at MGH, and a NIH T32 fellowship in Cardiac Imaging at MGH.
email: mlu@mgh.harvard.edu
Research Highlights
In Nature Comm, AIM scientists show that AI applied to X-rays can be used as a new biomarker source in cancer.
A recent publication validated a lung cancer prediction model in 14,737 patients from Mass General Brigham.
In this paper we demonstrate that deep learning applied to x-rays can be used as a new biomarker source.
In Nature Comm, we show that deep learning can automatically predict cardiovascular events.
We developed several heart segmentation algorithms that work on gated and non-gated CT scans.
An automated deep-learning approach based on chest x-rays can improve lung cancer screening.
JAMA Network Open published our study about using deep learning to extract prognostic information from chest radiographs