Simon Bernatz
Research Fellow
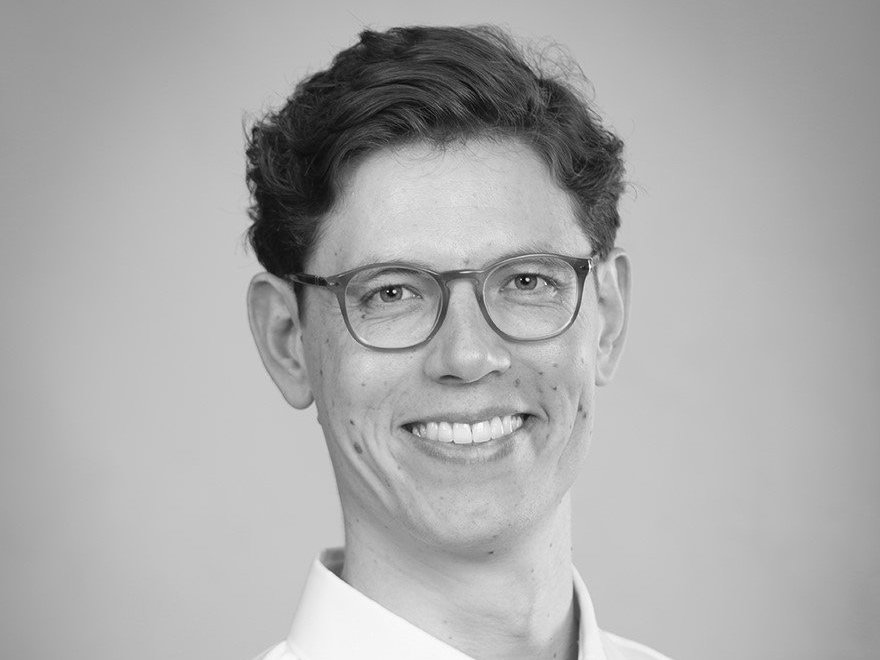
Simon Bernatz MD is a radiology resident and a research fellow at AIM as well as a visiting scholar at Harvard Medical School. He is currently enrolled in the PhD program at Maastricht University, the Netherlands at the Faculty of Health, Medicine and Life Sciences (FHML). His research interests are in the translational intersection of medical care, radiology, and data science with a focus on bridging the AI translational gap.
He is especially interested in analyzing the interpretability, usability, utility, and clinical relevance of medical AI applications with a focus on oncology and cardiology. With his research, he wants to leverage trust in AI applications, not only in the context of personalized precision medicine but also from a socio-economic and ethical point of view. He is enrolled in a Master’s Program in Health Business Administration, facing the global increase in tumor burden and healthcare costs, AI’s impact on medicine could lead to the development of time-/ cost-effective methods for biomarker evaluation, patient care, and stratified medicine.
He graduated from Goethe University Medical School, Frankfurt am Main, Germany. He earned his MD (Dr. med.) from Goethe University analyzing the impact of chemotherapy on the blood-brain barrier at the Edinger Institute. He has been awarded the Walter Benjamin Fellowship of the German Research Foundation (DFG) to conduct his research at AIM.
email: sbernatz@bwh.harvard.edu
Research Highlights
Researchers at AIM investigated the use of LLMs for patient portal messaging.
AIM Researchers build foundation model to discover new cancer imaging biomarkers
AIM study investigates if AI can highlight social determinants of health from clinical notes
AIM investigators developed AI to track muscle mass for children through young adulthood
AIM researchers investigate ChatGPT for its ability to provide cancer treatment recommendations
In Nature Comm, AIM scientists show that AI applied to X-rays can be used as a new biomarker source in cancer.
AIM investigators published a clinical evaluation of AI algorithms to screen for extranodal-extension on CT.
In Nature Medicine, AIM and TRACERx investigators show the importance of AI-based body composition.
A recent publication validated a lung cancer prediction model in 14,737 patients from Mass General Brigham.
We developed an AI model that can accurately predict distant metastases after treatment for lung cancer patients.
In Lancet Digital Health, we published a clinical validation of deep learning algorithms to target lung cancer tumors.
In this paper we demonstrate that deep learning applied to x-rays can be used as a new biomarker source.
In Cancer Cell, we published our perspective on the impact of AI in Clinical Oncology.
In Nature Comm, we show that deep learning can automatically predict cardiovascular events.
We developed several heart segmentation algorithms that work on gated and non-gated CT scans.
AIM investigators describe their view on AI reproducibility and transparency.
An automated deep-learning approach based on chest x-rays can improve lung cancer screening.
In The Lancet Digital Health, AIM investigators have defined the levels of autonomy in medical AI.
In Nature Reviews Clinical Oncology, we highlight how AI is transforming the field of radiation oncology to treat patients more accurately and efficiently.